Table Of Content

Like group designs with within-subject comparisons, single-subject designs can control for individual differences, which remain constant. Additionally, single-subject designs measure multiple data points through time within each condition before any experimental change occurs to assess pre-existing variation and trends in comparisons with the subsequent condition. Of course, a single-subject experiment without inter-individual replication has no generality—systematic replications across relevant individual characteristics and contexts are generally required to establish external validity. However, the typical group design also often requires similar replication to establish the same validity, and unlike group designs single-subject studies are also capable of rigorously interrogating even the rarest of effects. These designs require data from multiple individuals (people, animals, cells, etc.).
Multiple-Baseline and Multiple-Probe Designs
An excellent discussion of this issue can be found in the exchange of letters to the editor by Hoodin (1986) [Article] and Rubow and Swift (1986) [Article]. Single-subject studies should not be confused with case studies or other non-experimental designs. One of the biggest mistakes, that is a huge problem, is misunderstanding that a case study is not a single-subject experimental design.
Multiple-Treatment Designs
But if the dependent variable changes when the treatment is introduced for multiple participants—especially when the treatment is introduced at different times for the different participants—then it is extremely unlikely to be a coincidence. Because replication of the experimental effect is across conditions in multiple-baseline/multiple-probe designs, they do not require the withdrawal of the intervention. This can make them more practical with behaviors for which a return to baseline levels cannot occur. Depending on the speed of the changes in the previous conditions, however, one or more conditions may remain in the baseline phase for a relatively long time.
Precedent of within-subject methods
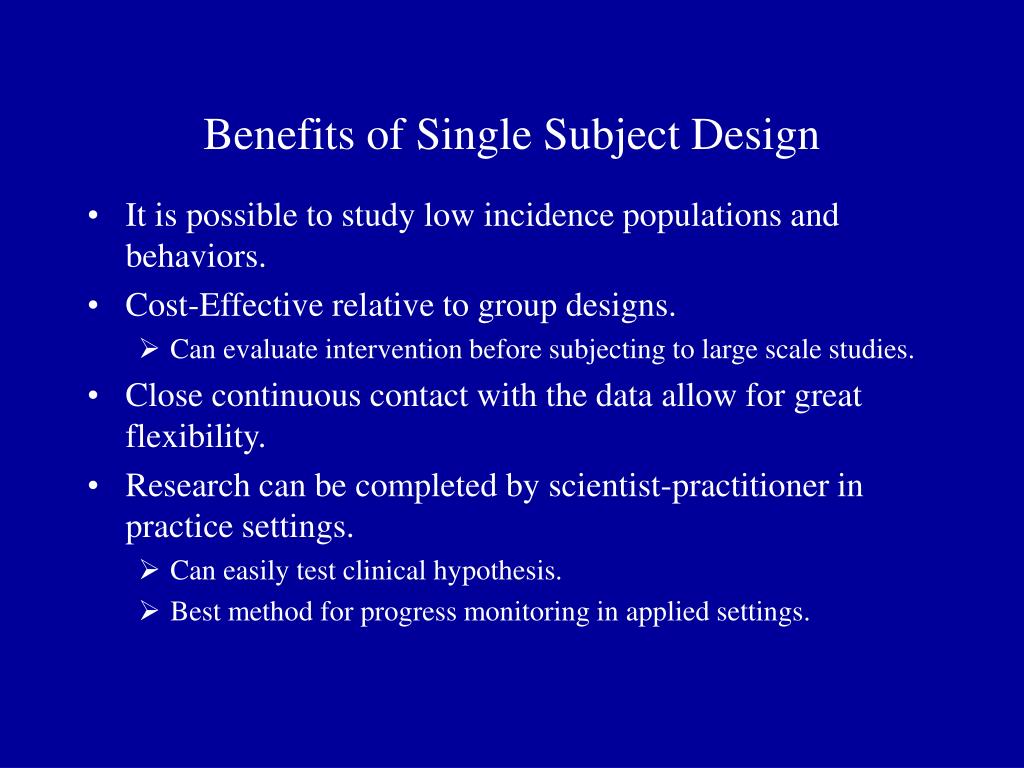
On the other hand, there is overlap between the baseline and intervention phases in Panel B of Figure 1, and the overall level of the dependent variable does not differ much between the phases. There is, however, a change in trend, as there is a consistent decreasing trend during the baseline phase, which is reversed in the intervention phase. In the current climate of evidence-based practice, the tools provided by SSEDs are relevant for researchers and practitioners alike.
Abnormal Technology: Definition, Topics, Criticisms - Verywell Mind
Abnormal Technology: Definition, Topics, Criticisms.
Posted: Wed, 10 May 2023 07:00:00 GMT [source]
Changing criterion design
Referred to as treatments that have substantial effects on important behaviors and that can be implemented reliably in the real-world contexts in which they occur. By focusing daily data collection on one participant, researchers can prepare to expand their research. This research method yields a high amount of data that can be analyzed by researchers. This data can then be used to support a researchers hypothesis and/or give insight before moving on to a group research project. When the researcher waits until the participant’s behavior in one condition becomes fairly consistent from observation to observation before changing conditions. A study method in which the researcher gathers data on a baseline state, introduces the treatment and continues observation until a steady state is reached, and finally removes the treatment and observes the participant until they return to a steady state.
What Makes Psychology a Science?→
So, if I were doing a group treatment study, I would not necessarily be able to see or to understand what was happening with each individual patient, so that I could make modifications to my treatment and understand all the details of what’s happening in terms of the effects of my treatment. An advantage of using an SSRD is that, instead of comparing the percentage of people that responded to an experimental factor to the percentage of people that did not, the study examines how an individual subject, with his own unique characteristics, responds to the experimental factor. This is particularly useful when studying specific subsets of a population, rather than the population as a whole.
This is because (a) the final two final treatment phases do not include the minimum of three data points and (b) the individual treatment component phases (FCT only and time-out/DRO) were implemented only once each. As a result, the data from this study could not be used to support the treatment package as an evidence-based practice by the IES standards. Additional data points within each phase, as well as replications of the phases, would strengthen the study results. When selecting conditions for a multiple-baseline (or multiple-probe) design, it is important to consider both the independence and equivalence of the conditions.
Interpretation of data
One reason is that case studies usually do not allow researchers to determine whether specific events are causally related, or even related at all. For example, if a patient is described in a case study as having been sexually abused as a child and then as having developed an eating disorder as a teenager, there is no way to determine whether these two events had anything to do with each other. A second reason is that an individual case can always be unusual in some way and therefore be unrepresentative of people more generally. Although within-subject group experiments are common in human neuroscience and psychology, e.g., Greenwald (1976) and Crockett and Fehr (2014), full-fledged single-subject designs are virtually unknown in many subfields. Still, high-impact neuroscience experiments have occasionally either implicitly or deliberately implemented within-subject reversals, demonstrating the power of these approaches to advance the science.
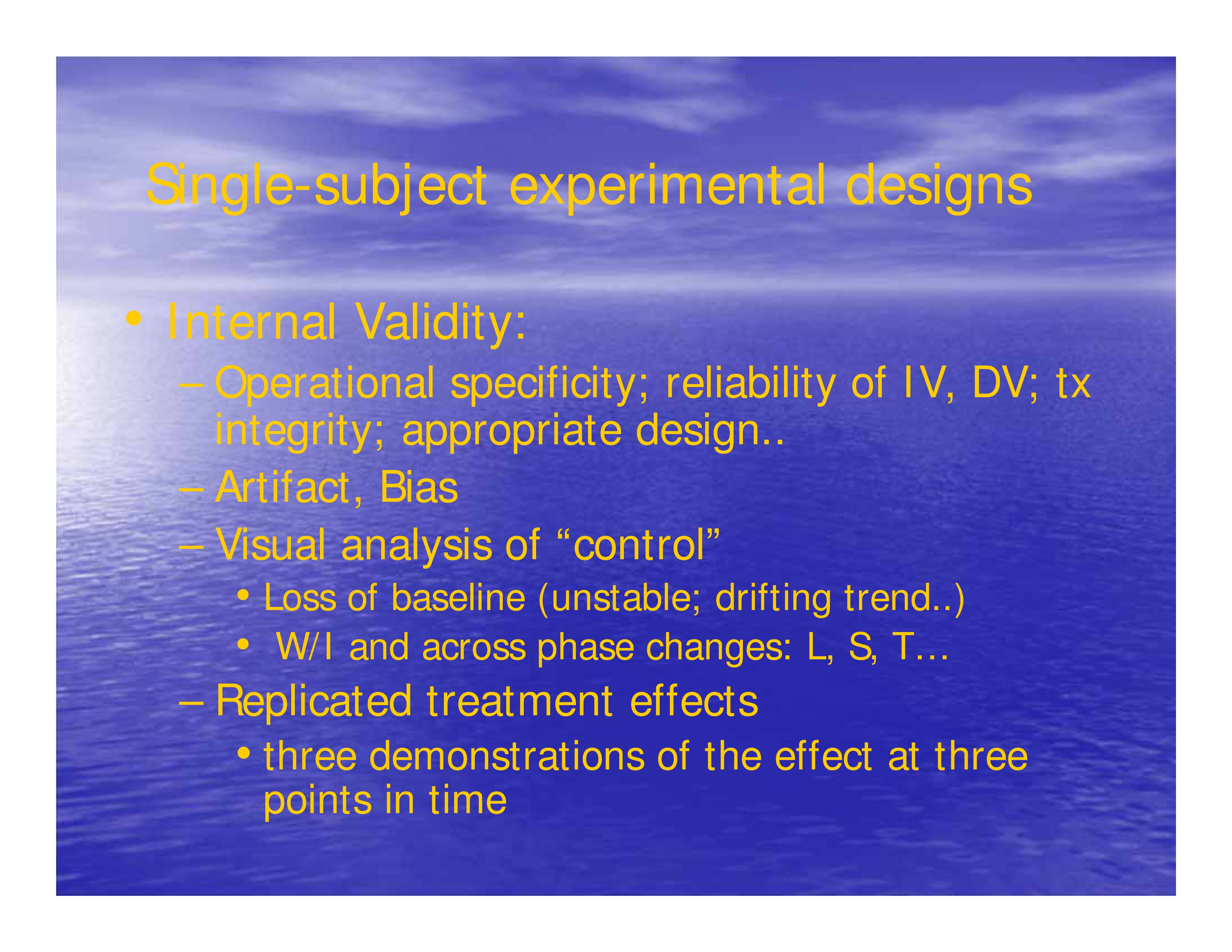
Withdrawal designs (e.g., ABA and ABAB) provide a high degree of experimental control while being relatively straightforward to plan and implement. However, a major assumption of ABAB designs is that the dependent variable being targeted is reversible (e.g., will return to pre-intervention levels when the intervention is withdrawn). If the individual continues to perform the behavior at the same level even though the intervention is withdrawn, a functional relationship between the independent and dependent variables cannot be demonstrated. When this happens, the study becomes susceptible to the same threats to internal validity that are inherent in the AB design.
But if the dependent variable changes with the introduction of the treatment and then changes back with the removal of the treatment (assuming that the treatment does not create a permanent effect), it is much clearer that the treatment (and removal of the treatment) is the cause. Single-subject research, at times referred to as single-case research, is a quantitative approach to examine functional relationships between baseline and experimental conditions over time within individual subjects. Collected observational data are converted to a standard metric and plotted in a line graph and visually analyzed to note variations in trend, level, and variability of the data across baseline and intervention conditions. Single-subject researchA type of quantitative research that involves examining in detail the behavior of each of a small number of participants.
The conflation of statistical methods with group designs has also led to the misconception that single-subject designs cannot be analyzed statistically. Most scientists have less familiarity with statistical analyses appropriate for use in single-subject designs and the serially-dependent data sets that they produce. While pronounced effects uncovered in single-subject experiments can often be clearly detected using appropriate visual analysis, rigorous statistical methods applicable to single-subject designs are also available (e.g., Parker and Brossart, 2003; Scruggs and Mastropieri, 2013). Although the clinical/educational appeal of such a metric seems obvious (easy to calculate, it is consistent with visual inspection of graphic data), there are potential problems with the approach.
Thus, consistent with all of the points made above, sound methodology (design, measurement) is the biggest determinant of valid decision making. Overall, the four issues discussed above—effect detection, magnitude of effect, quality of the inference, and practice decisions—reflect the critical dimensions involved in the analysis of SSED. The importance of any one dimension over the other will likely depend on the purpose of the study and the state of the scientific knowledge about the problem being addressed.
Rather than comparing between phases, all the data points within a condition (e.g., all sessions of Intervention 1) are connected (even if they do not occur adjacently). Demonstration of experimental control is achieved by having differentiation between conditions, meaning that the data paths of the conditions do not overlap. In the top panel of Figure 10.6, there are fairly obvious changes in the level and trend of the dependent variable from condition to condition.
The greater the percentage of nonoverlapping data, the stronger the treatment effect. Still, formal statistical approaches to data analysis in single-subject research are generally considered a supplement to visual inspection, not a replacement for it. The mean and standard deviation of each participant’s responses under each condition are computed and compared, and inferential statistical tests such as the t test or analysis of variance are applied (Fisch, 2001)[3]. (Note that averaging across participants is less common.) Another approach is to compute the percentage of non-overlapping data (PND) for each participant (Scruggs & Mastropieri, 2001)[4].
So, one instance of one treatment being implemented with one behavior is not sufficient, even if you’ve measured other things. In response, they note that the strong and consistent effects they are typically interested in—even when observed in small samples—are likely to generalize to others in the population. Single-subject researchers also note that they place a strong emphasis on replicating their research results.
A baseline phase is followed by separate phases in which different treatments are introduced. The dependent variable ranges between 10 and 15 units during the baseline, then has a sharp decrease to 7 units when treatment is introduced. However, the dependent variable increases to 12 units soon after the drop and ranges between 8 and 10 units until the end of the study. Research designs are traditionally preplanned so that most of the details about to whom and when the intervention will be introduced are decided prior to the beginning of the study. However, in single-subject designs, these decisions are often made as the data are collected.[10] In addition, there are no widely agreed-upon rules for altering phases, so conflicting ideas could emerge as to how a research experiment should be conducted in single-subject design. However, a closer look at individual results showed that 8 subjects actually decreased their frequency of stuttering, one didn't change, and 11 demonstrated an increase during the faster speaking condition.
No comments:
Post a Comment